Machine Learning Approaches to Classify and Predict Congenital Jaundice
DOI:
https://doi.org/10.69938/Keas.2401025Keywords:
Machine Learning, Categorization, Congenital jaundice, Model Evaluation, AUC-ROC AnalysisAbstract
Neonatal Jaundice, a common condition in newborns, results from elevated bilirubin levels, leading to the characteristic yellowing of the skin and eyes. Timely and precise classification and prediction of neonatal jaundice are crucial for early medical intervention. Machine learning has emerged as a powerful tool in healthcare for creating predictive models. This abstract offers an overview of machine learning methods used to classify and predict neonatal jaundice, incorporating clinical, laboratory, and genetic data. The dataset used in this research has been collected from patients at the Sulaimani Children's Hospital. The sample was collected over 5 months, from 2022 to 2023. Number of rows: 130 (one for each patient's data) There are 8 attributes in each record with Y as the target attribute consisting of jaundice levels The remaining seven characteristics are what the algorithm uses to predict. All characteristics are discrete. Table 1 / Dataset used in this study Full size table. Two types of machine learning algorithms are used: KNN and Naive Bayes. The assessment of model performance relies on metrics such as accuracy, sensitivity, specificity, and AUC-ROC, revealing encouraging outcomes. The results show that the classification of congenital jaundice using the KNN (k = 15) algorithm gives us accurate classification results when compared with the Naive Bayesian algorithm, and its classification percentage is equal to 64.34%.
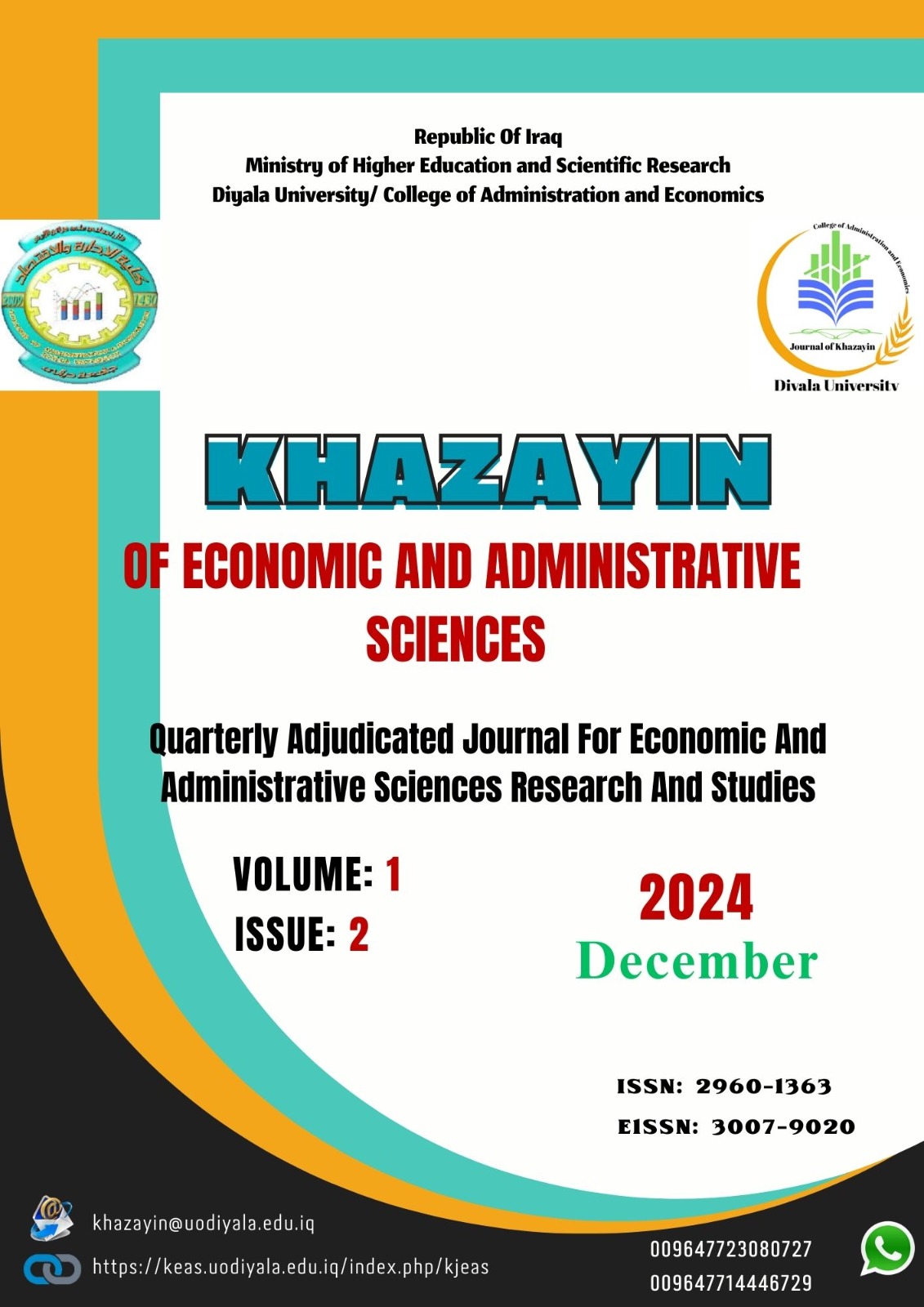
Downloads
Published
How to Cite
Issue
Section
License
Copyright (c) 2024 Khazayin of Economic and Administrative Sciences

This work is licensed under a Creative Commons Attribution-NonCommercial 4.0 International License.